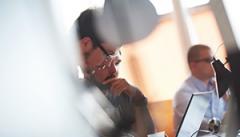
Sciences & Société
Soutenance de thèse : Corentin KERVADEC
Biais et raisonnement dans les systèmes de questions réponses visuelles
Doctorant : Corentin KERVADEC
Laboratoire INSA : LIRIS
Ecole doctorale : ED512 Informatique Et Mathématiques de Lyon
This thesis addresses the Visual Question Answering (VQA) task through the prism of biases and reasoning. VQA is a visual reasoning task where a model is asked to automatically answer questions posed over images. Despite impressive improvement made by deep learning approaches, VQA models are notorious for their tendency to rely on dataset biases, preventing them from learning to `reason’.
Our first objective is to rethink the evaluation of VQA models. Questions and concepts being unequally distributed, the standard VQA evaluation metric, consisting in measuring the overall in- domain accuracy, tends to favour models which exploit subtle training set statistics. We introduce the GQA-OOD benchmark designed to overcome these concerns: we measure and compare accuracy over both rare and frequent question-answer pairs, and argue that the former is better suited to the evaluation of reasoning abilities.
Evaluating models on benchmarks is important but not sufficient, it only gives an incomplete understanding of their capabilities. We conduct a deep analysis of a state-of-the-art Transformer VQA architecture, by studying its internal attention mechanisms. Our experiments provide evidence of the existence of operating reasoning patterns, at work in the model’s attention layers, when the training conditions are favourable enough. As part of this study, we design an interactive demonstration (available at https://visqa.liris.cnrs.fr/) exploring the question of reasoning vs. bias exploitation in VQA.
Finally, drawing conclusion from our evaluations and analyses, we come up with a method for improving VQA model performances. We explore the transfer of reasoning patterns learned by a visual oracle, trained with perfect visual input, to a standard VQA model with imperfect visual representation. Furthermore, we propose to catalyse the transfer though reasoning supervision, either by adding an object-word alignment objective, or by predicting the sequence of reasoning operations required to answer the question.
Additional informations
-
Orange Innovation (Rennes) - Lien pour assister à la soutenance => https://bit.ly/32SYxG5