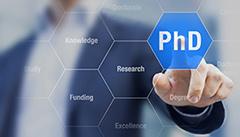
Sciences & Société
Soutenance de thèse : Matthieu LAURENDEAU
« Tomographic incompleteness maps and application to image reconstruction and stationary scanner design »
Doctorant : Matthieu LAURENDEAU
Laboratoire INSA : CREATIS
École doctorale : ED160 : EEA (Electronique, Electrotechnique, Automatique)
Computed tomography (CT) is one of the most commonly used modality for three-dimensional (3D) imaging in the medical and industrial fields. In the past few years, new X-ray sources have been developed based on carbon nanotube (CNT) cathodes. Their compact size enables the design of a new generation of multi-source CT scanners. In contrast to traditional systems with a single moving source, these scanners often adopt stationary architectures where multiple sources are static. It would benefit both industry with cheaper and motionless systems and medical applications with light-weight and mobile scanners which could be brought to emergency sites. However, this type of scanner uses a fewer number of measurements, known as projections, and may acquire data with a limited range of angles, leading to well-known image reconstruction challenges. This thesis focuses on the design of such stationary CT scanners. Three axes of study are investigated.
The first contribution is the development of an object-independent metric to assess the reconstruction capability of a given scanning geometry. Based on Tuy's condition, the metric evaluates local tomographic incompleteness and is visualized through 3D vector field maps. It is further extended to handle truncated projections, improving its applicability to real-world configurations. The metric enables ranking different geometries, predicting image quality reconstruction, and identifying the origin of geometric artifacts. It is applied to a variety of geometries, including existing scanners.
The second is a novel local regularization method to address limited-angle reconstruction challenges. The method employs a directional total variation (DTV) regularizer whose strength and directional weights are adaptively selected at each voxel. The weights are determined based on the previously introduced metric. Two approaches for directional weights are explored: ratio-based weighting relative to image axes and ellipse-based weighting. The reconstruction algorithm is evaluated in both 2D and 3D simulations, considering noiseless and noisy data, as well as real data.
The third is a tool for optimizing the geometry of CT scanners. Given a fixed number of sources and the surface area available for their positions, the tool optimizes the placement of sources based on the proposed metric. Several state-of-the-art optimization algorithms are implemented and tested on simple 2D and 3D scenarios.
Additional informations
-
Amphithéâtre de la délégation du CNRS Rhône Auvergne (Villeurbanne)