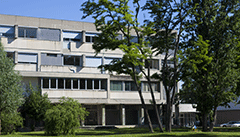
Sciences & Société
Soutenance de thèse : Safuriyawu AHMED
Resillient IoT-based Monitoring System for the Nigerian Oil and Gas Industry
Doctorante : Safuriyawu AHMED
Laboratoire INSA : CITI
Ecole doctorale : ED160 : Electronique, Electrotechnique, Automatique
Pipeline failures in crude oil transportation occur due to ageing infrastructure, corrosion, vandalisation, equipment failures among others. Consequently, hydrocarbons are released to the environment resulting in environmental pollution, ecological degradation, unprecedented loss of lives and revenue to mention a few. As a result, multiple leakage detection and monitoring systems (LDMS) are employed to mitigate failures in pipeline network. More recently, these LDMS include the use of Wireless Sensor Networks (WSN) and Internet of Things (IoT)-based system. While they are proven to be more efficient than other LDMS, many challenges exist in the adoption of such system for pipeline monitoring. These include, the fault tolerance, energy consumption, accuracy in leakage detection and localisation, high false alarms and so on.
Therefore, our work seeks to address some of the challenges in the implementation of IoT-based system for crude oil pipelines in an end-to-end manner. Specifically, we consider the aspect of accurate leakage detection and localisation by introducing a unique node placement strategy based on fluid propagation for sensitive and multi-sized leakage detection. Additionally, we propose a new distributed leakage detection technique (HyDiLLEch) in the wireless sensor network layer. It is based on a fusion of existing leakage detection techniques such as the negative pressure wave method, gradient- based method, and pressure point analysis. With HyDiLLEch, we efficiently eliminate single point of failure. Furthermore, we implement a fault-tolerant data and service management in the fog layer utilising the Nigerian National Petroleum Corporation (NNPC) pipeline network as a use case. The problem is modelled as a game against nature following the historical data of the incident rate in the NNPC pipeline. Our proposed regionalised solution (R-MDP) using reinforcement learning ensures the optimisation of accuracy and fault tolerance while minimising the energy consumption.
Información adicional
-
Amphithéâtre Est des humanités - Villeurbanne