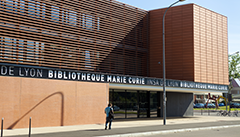
Sciences & Société
Soutenance de thèse : Yamil VINDAS-YASSINE
Weakly-supervised learning for emboli characterization with Transcranial Doppler (TCD) monitoring
Doctorant : Yamil VINDAS-YASSINE
Laboratoire INSA : CREATIS
Ecole doctorale : ED160 : Electronique, Electrotechnique, Automatique
This thesis focuses on the classification and characterization of high intensity transient signals coming from portable transcranial Doppler (TCD) ultrasound devices. The main objective is to help clinicians identify solid and gaseous emboli from artifacts generated during TCD monitoring sessions. In fact, emboli are solid or gaseous particles that can circulate in the cerebral arteries, sometimes blocking them and causing ischemic stroke. However, the identification and classification of HITS between solid embolus, gaseous embolus, and artifacts is not evident and require important expert knowledge. Therefore, its detection, classification and characterization are key factors to improve patient management in healthcare centers. In this work, we propose deep learning models capable of doing an accurate classification between solid embolus, gaseous embolus, and artifacts, with limited memory and energy consumption. More precisely, for HITS classification, to our knowledge, our work is the only one proposing an in vivo classification of portable TCD HITS between solid embolus, gaseous embolus, and artifacts. The main contributions of this work are the following. Firstly, we proposed a semi-automatic data annotation method based on local quality metrics with controlled annotation error, allowing to quickly label a large dataset, using a small number of labeled samples [1, 2]. Secondly, we propose a hybrid guided and regularized multi- feature classification model allowing to accurately classify HITS, simultaneously taking advantage of the raw Doppler signal, and its time-frequency representation [3, 4]. Finally, we proposed new model compression techniques based on pruning and extreme quantization, allowing to reduce the memory requirements of the trained models, as well as the energy consumption. Finally, as we worked in close cooperation with Atys Medical, manufacturer of portable TCD devices, we were able to incorporate our developed models into their data management software.
Información adicional
-
Amphithéâtre Emilie du Châtelet (Bibliothèque Marie Curie) - Villeurbanne